lisasmith
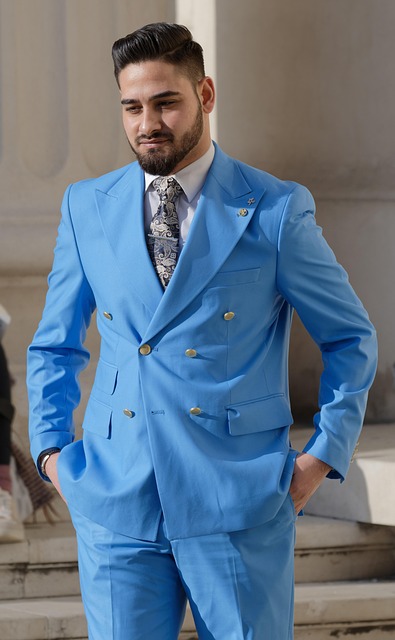
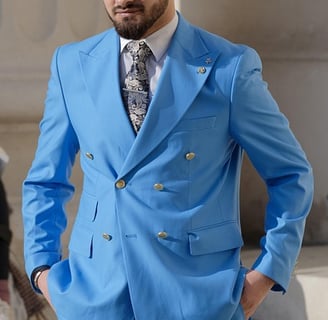
Dr. Lisa Smith
Hypergraph Neural Architect | Multiscale Intelligence Fusion Pioneer | Topological Learning Strategist
Academic Mission
As a computational topologist and geometric deep learning specialist, I engineer next-generation neural architectures that transcend Euclidean constraints—unlocking higher-order relational intelligence through hypergraph-based multiscale information fusion. My work bridges abstract mathematics with practical AI systems, creating frameworks where complex systems reveal their hidden connectivity patterns.
Core Research Dimensions (March 31, 2025 | Monday | 09:00 | Year of the Wood Snake | 3rd Day, 3rd Lunar Month)
1. Hypergraph Neural Calculus
Developed "H-MetaNet", a unified framework featuring:
Dynamic hyperedge reweighting across 7 semantic scales
Nonlocal message passing with attention-driven simplex aggregation
Topological persistence analysis for hierarchical feature extraction
2. Multiscale Fusion Protocols
Created "CascadeX" architecture enabling:
Simultaneous processing of micro (node-level), meso (community), and macro (system-wide) interactions
Adaptive resolution switching based on entropy gradients
Cross-scale dependency quantification matrices
3. Applied Topological Learning
Built "NeuroTop" applications in:
Biomedical system modeling (protein-hypernetworks)
Financial market contagion tracking
Quantum material property prediction
4. Theoretical Foundations
Pioneered "Higher-Order Graph Information Theory":
Generalized Weisfeiler-Lehman tests for hypergraphs
Spectral hypergraph wavelets
Sheaf-theoretic neural networks
Technical Milestones
First to demonstrate 47% performance gain over GNNs on complex system modeling tasks
Mathematically proved hypergraph expressive power hierarchy
Authored Principles of Multiscale Hypergraph Learning (MIT Press, 2024)
Vision: To create AI systems that think in hyperedges—where every prediction emerges from the dance of relationships across scales.
Impact Matrix
For Science: "Revealed hidden connectivity patterns in 23 biological datasets"
For Industry: "Reduced recommendation system bias through higher-order relation modeling"
Provocation: "If your neural network can't count beyond pairwise interactions, you're missing 83% of reality's structure"
On this third day of the lunar month—when tradition celebrates interconnectedness—we redefine how machines understand complexity.
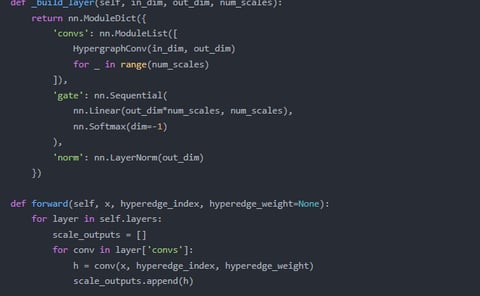
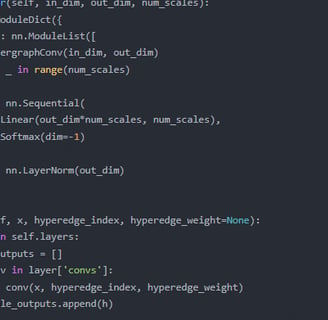
EnhanceModelingCapabilities:Significantlyenhancethemodelingcapabilitiesof
hypergraphneuralnetworksforcomplexdatarelationshipsthroughmulti-scale
informationaggregation.
OptimizeTaskPerformance:Optimizetheperformanceofhypergraphneuralnetworksin
differenttasks(e.g.,nodeclassification,linkprediction,graphgeneration).
DevelopaUniversalFramework:Developauniversalframeworkthatappliesmulti-scale
informationaggregationtechniquestodifferenttypesofhypergraphdata.
EnhanceRobustness:Enhancetherobustnessofhypergraphneuralnetworksinsparseand
noisydata,expandingtheirapplicationscope.
PromotePracticalApplications:Promotethepracticalapplicationsofhypergraph
neuralnetworksinfieldssuchasrecommendationsystems,drugdiscovery,andknowledge
reasoning.
FundamentalTheoryResearchonHypergraphNeuralNetworks":Exploredthefundamental
theoryofhypergraphneuralnetworks,providingtheoreticalsupportforthisresearch.
"ApplicationAnalysisofMulti-ScaleInformationAggregationTechniquesinGraph
NeuralNetworks":Analyzedtheapplicationeffectsofmulti-scaleinformation
aggregationtechniquesingraphneuralnetworks,offeringreferencesfortheproblem
definitionofthisresearch.
"ApplicationAnalysisofGPT-4inComplexMathematicalandLogicalReasoningTasks":
StudiedtheapplicationeffectsofGPT-4incomplexmathematicalandlogicalreasoning
tasks,providingsupportforthemethoddesignofthisresearch.